- MAIN PAGE
- – elvtr magazine – 6 Key Obstacles to AI/ML Adoption in FinTech
6 Key Obstacles to AI/ML Adoption in FinTech
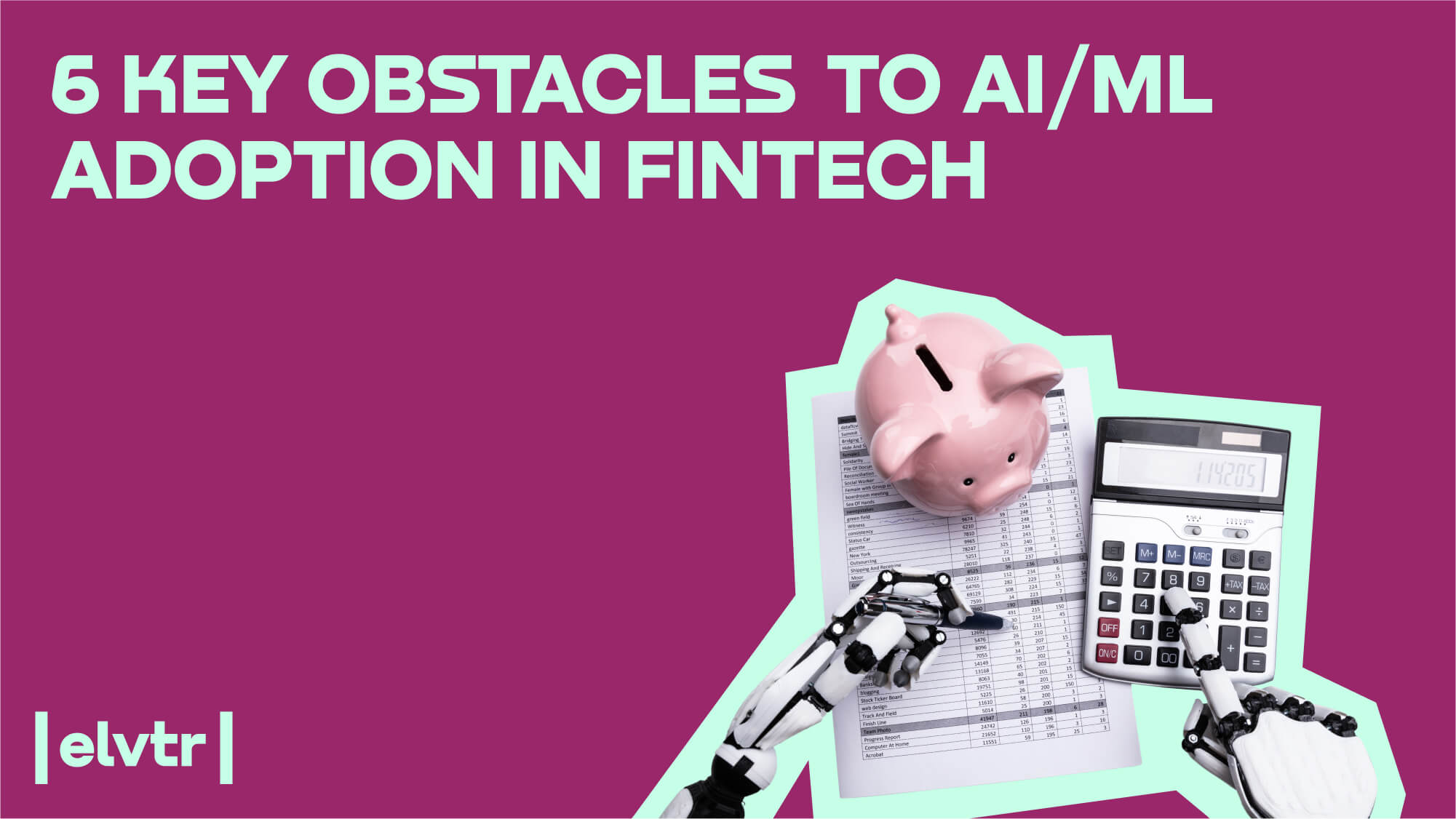
Since the COVID-19 pandemic, the fintech market has seen substantial growth, reaching a valuation of $305.7 billion in 2023. Emerging technologies such as machine learning and artificial intelligence are propelling the industry forward, introducing innovative solutions, setting new standards, and enabling fintech startups to compete with large tech corporations.
Remarkably, 80% of banks recognize the benefits of AI, and 85% plan to incorporate artificial intelligence in the development of new financial services. Despite these technologies proving immensely beneficial for the banking and finance sectors, certain challenges within the fintech industry still need addressing.
However, many AI projects are still in early stages and have not achieved full deployment, as noted in the Bank of England Machine Learning Survey. This blog post explores the primary obstacles to widespread AI adoption in the financial industry.
#1. Lack of Trust in AI & ML
Complex machine learning models are challenging to interpret or explain in terms of their predictions. Moreover, most people are unfamiliar with how AI functions. Without understanding, trust in AI output is hard to establish.
This was highlighted in a 2016 experiment at Virginia Polytechnic Institute and State University. Researchers sought to understand if humans and deep networks process tasks similarly. They showed a neural network a photo of a bedroom and asked what hung on the windows. Instead of focusing on the windows, the AI examined the floor first. After identifying the bed, the network deduced that blinds hung on the windows.
The neural network inferred the presence of blinds based on identifying the room as a bedroom. Though logical, this assumption overlooks that many bedrooms lack blinds.
The workings of advanced algorithms remain a mystery, driven by the behavior of thousands of artificial neurons across hundreds of layers. Consequently, financial service regulators and bankers, especially those involved in high-stakes decisions like fraud detection, find it hard to trust ML models. This challenge is amplified when transparency is crucial for preventing discrimination, ensuring fair outcomes, and fulfilling disclosure requirements.
#2. Talent Shortage
Implementing artificial intelligence projects requires a team of data professionals, including data scientists, analysts, engineers, and ML specialists. A Deloitte survey indicated that 23% of AI adopters identified a talent skills gap for AI implementation.
For example, a study by the Hong Kong Productivity Council (HKPC) found that 49% of AI companies struggle to hire AI-skilled talent. According to another research, one million additional ML & AI specialists will be needed by 2027. This shortage is especially pronounced in roles like data scientists, ML engineers, and AI developers.
Thus, the scarcity of data talent is a significant barrier to AI & ML adoption across various industries, including the finance sector. Bridging this skills gap necessitates a focused strategy for reskilling and upskilling specialized professionals.
Additionally, effective collaboration with universities, business incubators, and accelerators can attract top data and analytics talent.
#3. Lack of Data Standardization
AI and ML algorithms often receive financial data from diverse sources in various formats. While some algorithms can manage these inputs, standardized data allows for more efficient analysis and outcomes.
Hence, prior to integrating AI and ML solutions in enterprises, it's crucial to address:
- Data format variations due to differing currencies, date patterns, or accounting practices as per various country standards;
- Missing financial data, often resulting from incomplete records or privacy limitations;
- Time-dependency of financial information, such as stock prices, interest rates, or economic indicators, and more.
*Discover the gateway to the financial world for AI specialists! Learn to develop practical AI and ML solutions, from credit risk models to fraud detection, with SVP, Head of AI/ML Model Development for Consumer Lending at Wells Fargo, Paul J. Davis, through the ELVTR live online course on AI/ML in financial services.
#4. Regulatory Compliance
The financial sector, being highly regulated, must adhere to numerous laws and standards, including GDPR, GLBA, the Wiretap Act, and the Money Laundering Control Act. These ensure legal and transparent financial transactions and protect client rights and interests.
However, AI in finance presents new challenges that don't always align with existing regulations. AI's complexity, dynamism, and unpredictability make it hard to regulate and evaluate. Thus, developing a new legal and regulatory framework for AI in the finance sector is essential, considering its specifics, potential, and risks, and ensuring international consistency.
Additional measures for regulatory compliance include:
- Hiring an external legal consultant for guidance;
- Appointing an internal dedicated person or forming a department to monitor regulatory updates.
Using legal services is more cost-effective than risking GDPR penalties, which can reach up to €20 million.
#5. Data Security
AI in finance processes vast amounts of sensitive data, including personal customer information, financial records, bank accounts, and credit card details. This data is not only crucial for AI but also a target for hackers and scammers. A 2023 survey by Deep Instinct involving 650 cybersecurity experts revealed that 75% observed an increase in cybercrime, with 85% attributing this rise to AI exploitation by criminals.
Ensuring robust data protection against leaks, theft, and other threats is imperative. Companies should employ advanced encryption, authentication, anomaly detection technologies, and comply with confidentiality and consent rules for customer data processing, including rights to access, update, and delete personal data.
A case in point is the 2019 cybersecurity incident at Capital One, where an external party accessed and stole personal information of over 100 million customers and credit card applicants.
#6. Ethical and Liability Issues
AI in finance significantly impacts people's lives, influencing key financial decisions. Ensuring ethical and fair operation of finance AI and determining responsibility for its outcomes is vital. AI, like humans, can exhibit biases originating from algorithms or training data.
An ethical concern is AI's use in scoring and lending, potentially leading to unequal access to financial services or inaccurate customer creditworthiness assessments.
Thus, external control, supervision, and complaint mechanisms against finance AI are necessary. Enterprises must ensure transparency, explainability, and auditability in their operations.
McKinsey suggests six approaches for banking and financial companies and policymakers to address AI and bias.
AI in fintech is more than a trend; it's a transformative force in the financial sector. However, global companies need to develop and apply AI with awareness and expertise, considering its potential and risks, and adhering to standards that ensure reliability, transparency, fairness, and accountability.
*Interested in AI adoption in financial services? Join the live online course at the ELVTR platform. Utilize emerging technologies effectively, step into the finance industry as an AI expert, enhance your financial data analysis skills, and advance your career!