- MAIN PAGE
- – elvtr magazine – YOU CAN'T AFFORD TO WAIT ON AI: THE IMPACT OF ARTIFICIAL INTELLIGENCE IN BANKING
YOU CAN'T AFFORD TO WAIT ON AI: THE IMPACT OF ARTIFICIAL INTELLIGENCE IN BANKING
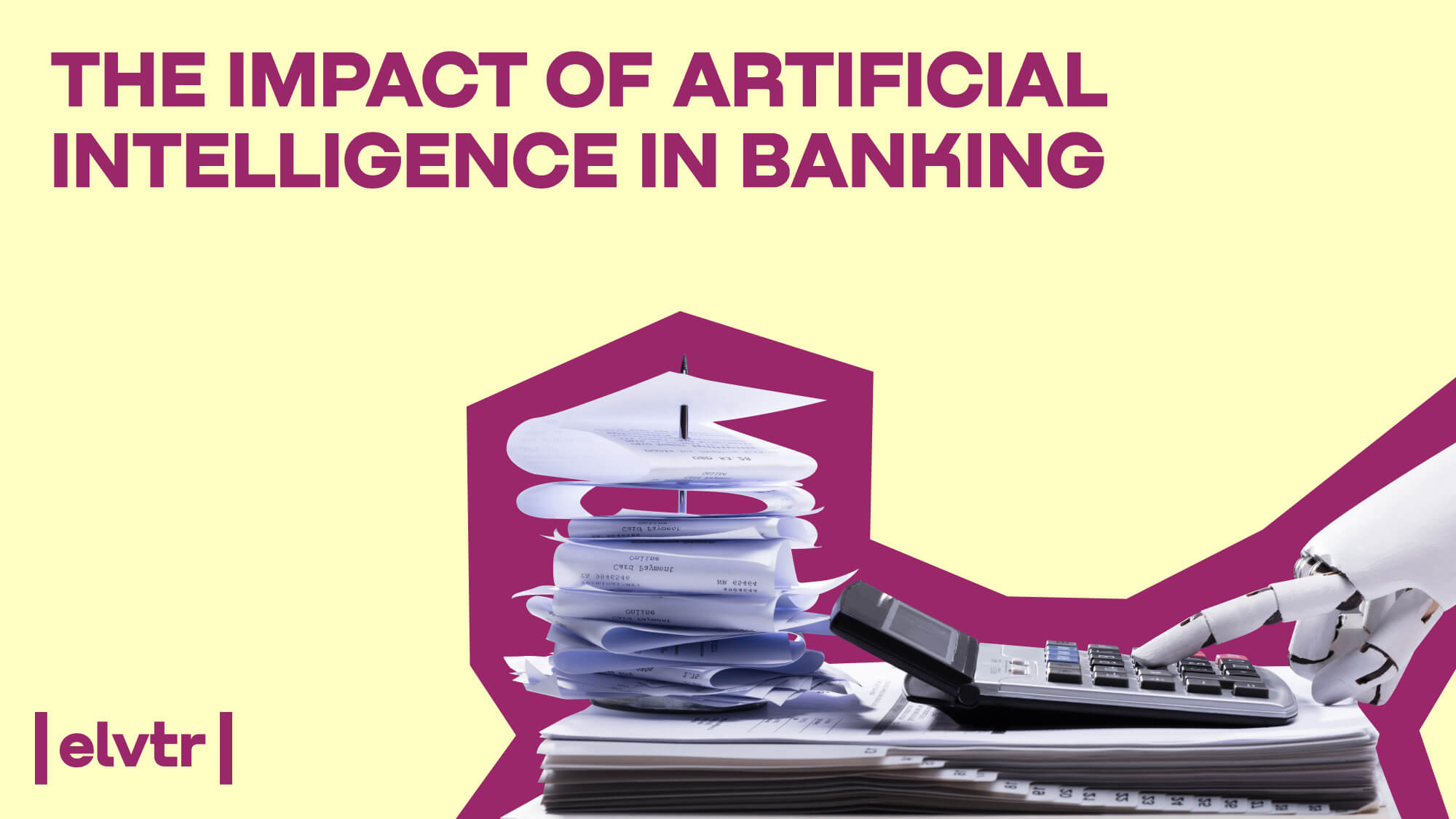
The rapid advancement of technology has been undeniable. Over the last year, AI showcased its capabilities, rivalling human aptitudes in perception, understanding, communication, creativity, reasoning, and application.
Artificial intelligence is poised to redefine entire industries, with banking being no exception. Business Insider indicates that nearly 80% of banks recognize the potential advantages of AI for financial services. McKinsey's research suggests that AI technologies could potentially unlock up to $1 trillion in additional value annually.
Here, we shed light on the significant opportunities and advantages of AI in banking and finance, ranging from investment strategies to tackling financial crime.
Top Benefits of AI for Financial Services
#1. Enhanced Customer Experience
Customer experience can either catapult a business to success or lead to its downfall. A staggering 86% of customers will abandon a trusted company after one or two subpar experiences. Moreover, 81% of organizations, including those in banking, identify customer experience as a key differentiator.
Challenges in banking customer experience can manifest as prolonged waiting periods, inadequate support, lagging websites, and less-than-stellar interactions with representatives. Deploying artificial intelligence in finance can ameliorate some of these challenges.
For example, chatbots can revolutionize customer support in the banking sector. Available around the clock, they respond to customer queries with a semblance of human interaction.
Responding instantaneously, users receive timely support, guidance in financial dealings, advice on services, savings plans, insights on expenditure, and deposit alternatives. Leveraging AI-driven assistants in FinTech could save banks an impressive $7.3 billion and 862 million labor hours globally by 2023.
#2. Refined Loan and Credit Decisions
Inappropriate loan and credit decisions rank among the leading causes of financial institution insolvencies. Through AI, banks can better gauge a customer's creditworthiness, enabling loan officers to make informed decisions. AI-powered platforms can scrutinize a client's credit past, transaction patterns, income, and employment tenure to predict their reliability in meeting debt obligations.
#3. Bolstered Security
The Association of Certified Fraud Examiners reports a staggering annual loss of over $3.5 trillion to fraud across businesses. The finance sector isn't immune. Fraudsters, now tech-savvier, employ sophisticated tools like machine learning.
Traditional fraud detection methods, reliant on manually crafted rule engines, identify just a fraction of fraudulent activities. Hence, financial bodies are rapidly adopting AI systems, with a whopping $217 billion investment in AI applications targeting fraud prevention and risk evaluation.
AI fortifies banking security by real-time transaction monitoring, fraud detection, and highlighting dubious transactions.
#4. Proficient Market Trend Analysis
In banking and finance, artificial intelligence empowers entities to process vast data sets, anticipating the latest market movements. Sophisticated machine learning methodologies assist in gauging market sentiments and proposing investment avenues. AI-driven solutions also pinpoint the optimal stock investment periods and raise alarms against potential risks.
#5. Amplified Efficiency
AI can streamline monotonous tasks like loan underwriting, data collation, report generation, compliance surveillance, and fraud spotting. This optimization allows staff to redirect their focus to intricate, strategic, and revenue-generating responsibilities. Projections indicate that AI will automate 44% of recurring finance tasks using chatbots and similar tools within three years.
Progressive FinTech companies recognize this potential. As per a Gartner study, almost 80% of finance leaders have either adopted or are contemplating the integration of AI-empowered automation tools.
*Delve into the ELVTR online course and harness real-world AI and machine learning applications in finance, spanning from credit risk modeling to fraud detection, guided by Wells Fargo's Head of AI Model Development, Paul J Davis.
Recommended courses
In-Depth Examples of Artificial Intelligence in Digital Banking
JPMorgan Chase: In 2014, a severe data breach hit JPMorgan Chase, impacting 76 million households and 7 million SMEs. Culprits extracted names, addresses, phone details, and emails of users across platforms like Chase.com, JPMorganOnline, Chase Mobile, and JPMorgan Mobile.
In response, by 2019, JPMorgan Chase's researchers engineered an AI-backed system using deep learning to identify malware, trojans, and phishing threats. Typically, a trojan can infiltrate company networks in about 101 days. This tool provides preemptive warnings, notifying the cybersecurity team even as cybercriminals gear up to dispatch malicious emails targeting the company's network.
Capital One: Capital One's innovative AI assistant, Eno, converses with users via text, offering account insights and facilitating credit card payments through smartphones. Clients might prompt Eno to display recent account balances or settle a credit card bill. Additionally, the technology safeguards online shoppers by generating temporary card numbers.
Bank of Latvia: Everyday, numerous customers approach the Bank of Latvia's consultants with queries about collectible coin availability, prevailing exchange rates, or cash operations. Their virtual assistant, Mona, furnishes 24/7 support. Remarkably, 30% of consultations occur post the standard working hours. Since its inception, Mona has advised over 2,000 clients, averaging 40 interactions daily and fielding about 25 calls typically meant for customer service representatives. This AI integration has lightened the bank's customer service burden by a quarter.
Mastercard: Mastercard harnesses AI and machine learning in finance for fraud detection and unauthorized transaction prevention. Their system, named Decision Intelligence, employs AI algorithms for transaction data analysis, spotlighting fraudulent patterns. This initiative enabled Mastercard to triple their detection of fraudulent activities and slash false positives by a factor of ten.
Danske Bank: Danske Bank, a dominant Nordic bank, exemplifies the use of deep learning and AI in fraud detection. Initially, the bank grappled with a mere 40% fraud detection rate and had to deal with up to 1,200 false alarms daily. Astonishingly, 99.5% of the cases under scrutiny turned out to be non-fraudulent. Addressing these false alerts drained significant resources in terms of manpower, hours, and funds. However, post AI-integration, Danske Bank reported a 60% dip in false positives, aspiring to reach an 80% reduction, while also improving true positive detections by 50%.
Wells Fargo: Traditionally, lenders relied on linear and non-linear regression models for financial forecasting and employed logistic and survivability models for default risk assessment. However, the advent of AI, machine learning, and deep learning is reshaping risk prediction.
Wells Fargo's novel system, dubbed "The Trifecta", utilizes machine learning algorithms to sift through customer data, identifying potential hazards. It evaluates a client's ability to service a loan. If a loan application gets declined, bank personnel can cite a specific code explaining the refusal. This innovation has bolstered the bank's risk management prowess, minimizing defaults on loans and credit cards.
*Dive deeper into the world of AI with ELVTR's course and explore its profound implications on the future of finance. Remember, staying ahead in the finance realm means not only understanding the numbers but also the technology that drives them.